Tools
Our Digital Public Goods empower communities and build resilience
All the components mentioned below are being integrated into the CommonsConnect platform for communities to understand the socio-ecological situation of their landscape, plan for the creation and repair of various natural resource management assets, and share these demands with relevant government programmes and donors for action. Watch this demo of a beta-version of the platform. Various geospatial layers will soon be made available for generation and download via a Google Earth Engine application.
Git-hub repo: https://gitlab.com/corestack.org/corestack
Intra-annual land use and land cover classification
Our research group has already made good progress in intra-annual LULC classification to determine areas under single cropping (one crop planted in a year, in the Kharif season) or multiple cropping (more than one crop planted in a year, in the Kharif and Rabi seasons), and other more static classes such as forest cover, surface water, and built-up areas. The figure below shows sample output over a few years. These LULC outputs will be used to compute evapotranspiration changes, canopy interception loss, runoff, etc., to construct a time series of groundwater changes.
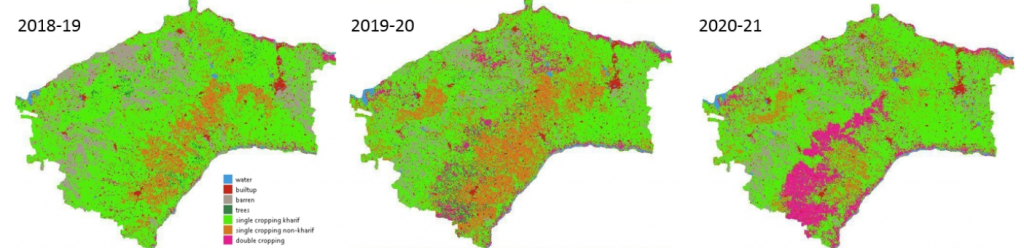
Building A Vulnerability And Socioeconomic Index
We are examining historic social welfare allocations made across hamlets and villages in the intervention panchayats to identify cases of unfair exclusion and assist the government in formulating vulnerability-proportional welfare allocation to these locations. We sre also obtain accessibility data of social infrastructure such as health facilities and schools from Google or Open Street Maps or data published by the government on the National Data and Analytics Platform (NDAP). Socio-economic factors proxied using a wealth index can be accurately obtained from classifiers trained on satellite-based data. The figure below shows such a sample output from the initial work by our group on poverty mapping. Social welfare expenditures will be obtained from common welfare schemes’ Management Information System (MIS) websites. Detailed health indicators have been estimated through co-variate modelling of coarser-level survey data at the district level, allocated to finer spatial scales.
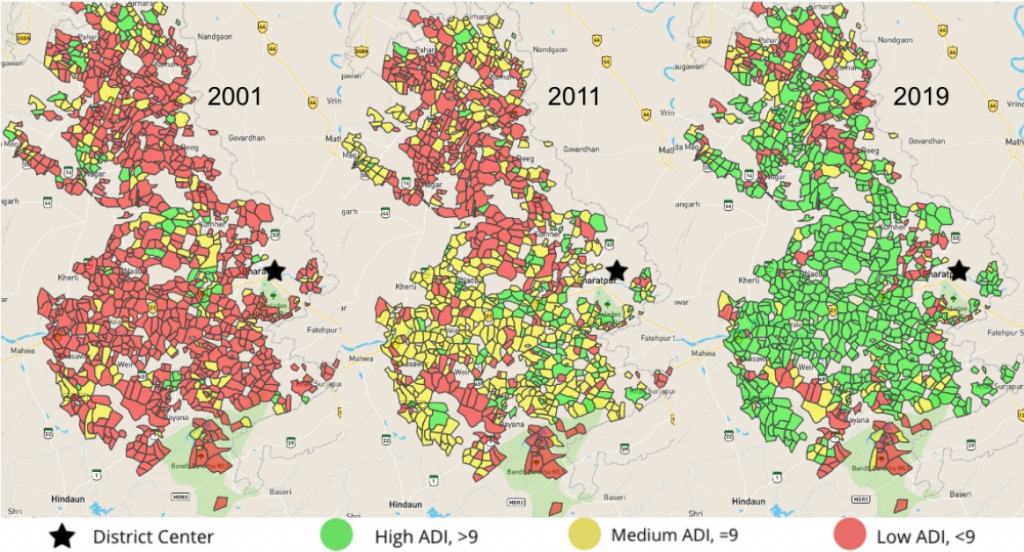
Landscape-Scale Planning Of Water Structures
Rainwater harvesting site assessment, for example, considers factors such as the soil type, slope, precipitation, soil moisture, land cover and land use. Remote-sensing data products of digital elevation maps and precipitation are now freely available. Machine learning-based land-cover classification algorithms that operate on freely available satellite imagery can provide high-resolution accurate maps, rapidly updatable. Soil moisture can similarly be estimated based on land surface temperature and vegetation indexes. We feel it is, therefore, feasible to conceive of a process whereby such datasets are used to identify candidate rainwater harvesting sites, fairness-based prioritizations are made to ensure the rights of vulnerable communities and participatory methods are then used to obtain community feedback and consensus to demand fund allocation for the identified sites.
Research Paper
Coming soon….
GitHub-Repository
Coming soon….
Community Forest Governance Tools
Geospatial digital applications for forest governance that community members can use to manage their forests better. Forest health is being mapped in high resolution using machine learning on satellite data, which shows vegetation density and open patches resulting from deforestation. Local insights are being added to build rules for the rotational harvesting of forest produce. Hydrological modelling is combined with these maps to recommend locations for new water management structures, contributing to improved forest growth. A geo-tagged incident database is being created to track forest fires, governance violations, corrective measures, and biodiversity, serving as a learning tool for local and other communities and contributing to citizen science databases for climate change.
Research Paper
Coming soon….
GitHub-Repository
Coming soon….
Impact Assessment
The main objective of this research is to quantitatively assess the impact of the water based NRM interventions undertaken by the government scheme Mahatma Gandhi National Rural Employment Guarantee Act (MGNREGA).
Remote-sensing techniques are being used to monitor pre-post indicators, such as vegetation index, groundwater level, and cropping intensity. This is done to develop a causal analysis framework to understand the change in outcome indicators.
We envision a tool that will provide an estimate of the impact of NRM assets and a projected impact for prospective NRM sites. Machine Learning (ML) models learned on the past farm ponds can probably be used to predict the site assessment for new prospective farm pond locations, and we will explore this next.
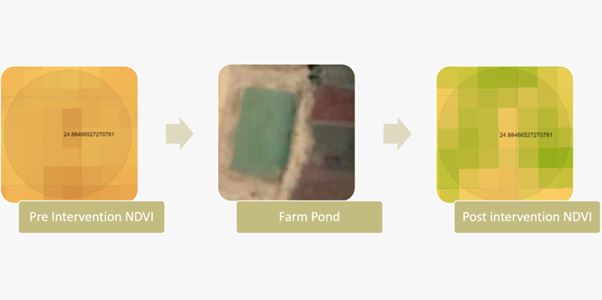
Research Papers
Coming soon.
GitHub-Repository
Coming soon.
How To’s
Coming soon.
Fairness In Funds Allocation
The objective of this research is to conduct an audit of the fairness in fund allocation in National Rural Employment Guarantee Act (NREGA) scheme at the panchayat level. The focus is on identifying whether there exists any discrimination against Scheduled Castes (SC) and Scheduled Tribes (ST) communities in the provision of employment opportunities under the scheme, despite them being vulnerable and the primary beneficiaries of the scheme. Preliminary experiments showed some instances of discrimination against these communities which motivated us to dive deeper into this.
Additionally, the study seeks to examine whether historically discriminated panchayats that have received lower funding in the past continue to receive less funding over time, or whether changes in political leadership or other factors have resulted in more equitable allocation of funds to these areas.
Ultimately, the research aims to develop a model to estimate optimal allocation of funds to promote equitable development among the panchayats. The model will take into account various factors such as the socio-economic and demographic characteristics of each panchayat, its developmental needs, and historical funding patterns to ensure that the allocation of funds is fair and just.
Research Papers
Coming soon.
GitHub-Repository
Coming soon.
How To’s
Coming soon.
Landscape Level Planning Of Tree Plantation
This research work focuses on building a participatory tool for assessing the land suitability for tree plantations and further recommend the best suitable tree species for the selected site that caters to the community’s environmental & economical needs. The project uses landscape characteristics like land use land cover, soil type, slope, elevation, proximity to nearest water body and other agro-climatic information of the region to generate the plantation feasibility and species recommendation map. The data for all these characteristics are derived by processing the high resolution open-source satellite imageries using the remote sensing and machine learning techniques.
We are also building a model to assess the plantation’s health over time, by estimating the vegetation density, growth in canopy height and carbon sequestered with time. This would facilitate the farmers, NGOs, government officials and other organizational bodies to analyze the impact of the undertaken NRM tree plantation projects in different places. We envisage to incorporate the learnings from the past plantation projects’ impact in the locality as well as the feedback from the on-ground users constantly into the tool to improvise the tree plantation planning.
Research Papers
Coming soon.
GitHub-Repository
Coming soon.
How To’s
Coming soon.
Smartforms: Optical scanning of paper forms for quick digitization of data and on-boarding of users
Data collection through digital devices may not always be feasible for reasons such as the unaffordability of smartphones and tablets by field-based cadre or shortfalls in training and capacity building. Paper-based data collection has been argued to be more appropriate in several contexts, with automated digitisation of the paper forms through OCR (Optical Character Recognition) and OMR (Optical Mark Recognition) techniques. We contribute by providing a large dataset of handwritten digits, and deep learning-based models and methods built using this data, that are effective in real-world environments.
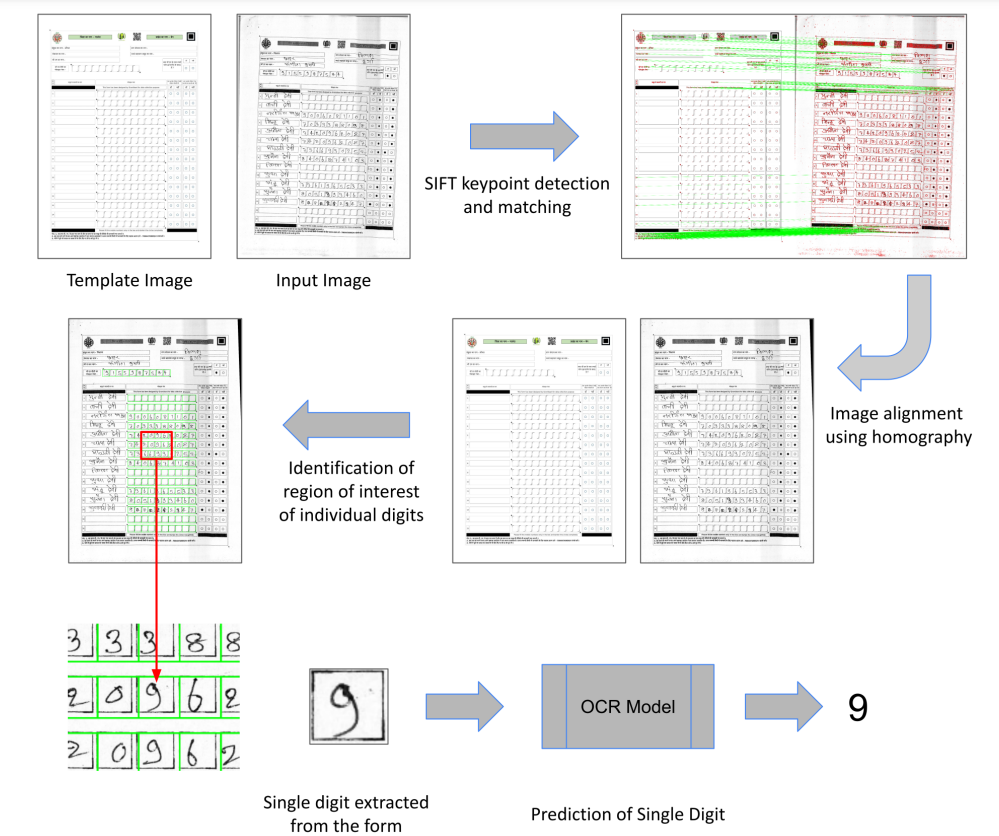
Research Papers
Use of Metric Learning for the Recognition of Handwritten Digits, and its Application to Increase the Outreach of Voice-based Communication Platforms – D. Pant, D. Talukder, D. Kumar, P. Pandey, A. Seth, and C. Arora.
ACM COMPASS, 2022.
GitHub-Repository
https://github.com/Smartforms2022/Smartforms
How To’s
Mobile Vaani: Voice-based community media
The IVR channel is popular among rural and low-income groups, while an app and WhatsApp messenger are available for smartphone users. Voice messages are moderated by local volunteers and Gram Vaani’s team. Acceptable messages are published on the platform for others to hear. Each platform is independent and coordinated by local volunteers who assist with social entitlements and public services. Incentives motivate volunteers to contribute to community development and acquire skills. Mobile Vaani has a strong reputation for providing credible information and assisting marginalised groups.
Research Papers
Design Lessons from Creating a Mobile-based Community Media Platform in Rural India – A. Moitra, V. Das, A. Kumar, Gram Vaani, and A. Seth.
ICTD 2016.
Findings from a Civil Society Mediated and Technology Assisted Grievance Redressal Model in Rural India – D. Chakraborty, S. Ahmad, and A. Seth.
ICTD, 2017
Costs and Benefits of Conducting Voice-based Surveys Versus Keypress-based Surveys on Interactive Voice Response Systems – A. Khullar, P. Hitesh, S. Rahman, D. Kumar, R. Pandey, P. Kumar, R. Tripathi, Prince, A.A. Jha, Himanshu, and A. Seth.
ACM COMPASS, 2021.
Experiences with the Introduction of AI-based Tools for Moderation Automation of Voice-based Participatory Media Forums – A. Khullar, P. Panjal, R. Pandey, A. Burnwal, P. Raj, A.A. Jha, P. Hitesh, R.J. Reddy, Himanshu, and A. Seth.
India HCI, 2021.
Early Results from Automating Voice-based Question-Answering Services Among Low-income Populations in India – A. Khullar, M. Santosh, P. Kumar, S. Rahman, R. Tripathi, D. Kumar, S. Saini, R. Pandey, and A. Seth.
ACM COMPASS, 2021.
GitHub-Repository
https://gitlab.com/gramvaani/mobilevaani
How To’s
Farmphone
FarmPhone technology solution will be built on top of the Mobile Vaani platform, an open-source enterprise resource planning system (customised for FarmPhone), and WhatsApp enabled front end for connecting customers.
- Mobile Vaani platform shall offer a dedicated bidirectional IVRS communication channel for farmers and Farmers’ organisations (Co-operatives, FPOs etc.). The platform shall connect farmers over mobile phones (feature and smartphones) to provide access to market information, knowledge, and post-harvest activities supported by Cooperatives. The platform shall be enabled with speech2text protocols to enable them to share their produce-related details in vernacular languages.
- An open-source ERP system to enable cooperatives to register the sale requests, improve traceability/transparency in the value chains, set up processes for procurements, track transactions and build health indicators for cooperatives – profits realised, corpus size, corpus utilisation. The functionality of Cooperative ERP:
- Farmers (sellers) services – IVR and WA channels register their produce to sell.
- Registration request for selling – IVR based survey for selected crops: type of produce, quantity available, and quality (optional)
- One-time registration through – ODK/Web-based registration by coop, WA, IVR (limited info), validation of registration by coop (Mobile Number, location, farmer registration number) for future transactions (transaction receipts, payment receipts)
- Communication with the farmer – IVR (Interactive Voice Response) system to push advisory messages to farmers, surveys, announcements, federation meeting minutes, and also for farmers to ask questions
- Verification, transport and selling transactions – Web mobile
- Registered request by farmers – notify or view all the sell requests, including details of a farmer, produce for verification and confirmation of the type, quantity and quality
- Procurement confirmation to coop – Sale receipt shared over SMS or WA
- Payment to farmers – Payment status options: Full advance payment made, partial advance payment made, commodity picked up, full payment made after delivery, etc. Sharing payment details over SMS or WA shall also be explored.
- Buyers (bulk buyers/local shops) – WA-based service (Primary),
- View the catalogue of bulk produce (unpackaged) – including product name, product type, quantity and price
- Registered buyers can get product notifications from Coop via WhatsApp and order on WA or IVR calls. This is a functionality for the front-end ERP for products.
- Farmers (sellers) services – IVR and WA channels register their produce to sell.
- WhatsApp enabled frontend – Augmenting the frontend with Whatsapp for consumer order placement. Additional channel for smartphone users/customers to explore the available products and place orders. Registered buyers can get notifications on products available from Coop via WhatsApp and can connect with Cooperatives over WA or IVR calls for purchasing commodities in bulk quantities.
Research Papers
Coming soon.
GitHub-Repository
Coming soon.
How To’s
Coming soon.
Commodity price forecasting application
Small and marginal farmers often find it hard to get a good price for their produce because of several logistical and contextual challenges they face in market participation. Aggregating produce via farmer cooperatives and being able to time the sales for non-perishable crops when market prices are high has emerged as a useful strategy for farmers. In our study, we work with a network of farmer cooperatives in India growing soybeans and explore the potential of developing a machine learning-based price forecasting and sales recommendation system that produces suggestions on the best dates for soybean sales should be made. Our expectation is that such a tool will provide useful indicators to farmer cooperatives on whether to sell their stored produce right away (if prices are likely to fall in the future) or to wait (if prices are likely to rise).
We present an evaluation of different methods for price forecasting and a prospect theory-based method to produce sales recommendations. Experiments on historical data indicate that we can provide modest gains to farmers, and we build and field test an Android application in collaboration with our field partners. Early results indicate positive feedback. Our methods can be generalized beyond soybean to other agricultural commodities that can be stored for several months and help farmer cooperatives to compete effectively in agricultural markets.
Research Papers
https://dl.acm.org/doi/full/10.1145/3609262
GitHub-Repository
https://github.com/souraavv/ai-based-market-intelligence-system
How To’s
Coming soon.